The prevalence of artificial intelligence (AI) tools in the field of science is on the rise, with a Nature survey of over 1,600 researchers globally indicating that many scientists foresee these tools playing a central role in the research process in the near future.
This article delves into the fascinating synergy between AI and scientific progress, illuminating how this collaboration is revolutionizing traditional approaches and opening doors to novel insights in a variety of scientific domains.
The Current Landscape and The Rise of AI in Science
In today's scientific landscape, researchers face both excitement and challenges. Traditional methods, involving meticulous data collection and manual analysis, have long been foundational to knowledge acquisition. However, the modern scene is marked by an unprecedented data influx, posing challenges in analysis, interpretation, and efficiency. Artificial Intelligence (AI), a technological revolution reshaping scientific exploration, proves to be a promising ally in overcoming challenges. Its rapid data processing, pattern recognition, and predictive modeling capabilities facilitate breakthroughs in data analysis, accelerating the pace of discovery across scientific domains. AI's application goes beyond analysis, automating repetitive tasks and allowing researchers to focus on creative problem-solving. The evolving synergy between AI and scientific progress has the potential to transform methodologies, accelerate discoveries, and usher in a new era of innovation. This represents not only a technological evolution, but also a paradigm shift in research approaches, promising ground-breaking discoveries in the years ahead.Potential Challenges and Limitations of AI in Scientific Research
Incorporating Artificial Intelligence (AI) in scientific research has many advantages, but it also has some potential challenges and limitations. The following are the current limitations and challenges of AI in Scientific Research:- Current statistical ML approaches require large amounts of data, which are often unavailable in science
- Many data sources must be annotated and labeled to be useful.
- Specialized scientific databases struggle with symbolically representing data for ML calculations while aligning with mathematical operations on data arrays.
- Common AI approaches like statistical machine learning (ML) and deep learning struggle with rational reasoning, abstract modeling, and logical inferences.
- Science is more concerned with accumulating knowledge and uncovering causal explanations than with classification tasks.
- In AI, bias often stems from the training data labeled by humans.
- Statistical ML operates differently from the human mind.
- Statistical ML is limited in its capacity for symbolic systems.
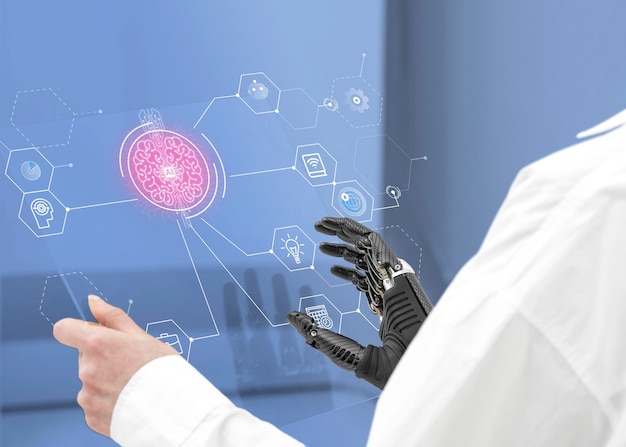
The Future of AI in Scientific Research
The future of AI in scientific research promises transformative advancements, shaping the landscape of inquiry. Key aspects include:- Accelerating discoveries through rapid data processing
- Enhancing data analysis for nuanced understandings
- Fostering interdisciplinary collaboration
- Automating repetitive tasks with AI-driven robotics
- Emphasizing ethical AI integration
- Augmenting scientific creativity
- Expanding personalized medicine
- Prioritizing explainable AI models, evolving AI technologies
- Promoting global collaboration and accessibility.